Personalized anticancer drug selection following companion diagnostic platform with customized biomarkers.
– As a cancer researcher, the use of circulating tumor microclusters (CTM) in companion diagnostics and personalized anticancer drug selection represents a groundbreaking advancement in oncology. CTM, derived from circulating tumor cell (CTC) organoid models, provide a unique opportunity to study the mechanisms underlying metastasis, including their ability to survive in circulation, evade immune detection, and colonize distant tissues.
– By integrating CTM analysis into diagnostic workflows, we can gain real-time insights into the dynamic changes in cancer cells during treatment. This enables the identification of biomarkers and the selection of personalized anticancer therapies that are optimally tailored to the patient’s specific tumor profile. Such approaches are particularly valuable in advanced or metastatic cancers, where tumor heterogeneity and adaptability often complicate treatment. Leveraging CTM for both diagnosis and therapeutic decision-making has the potential to improve clinical outcomes and open new avenues for targeting cancer metastasis
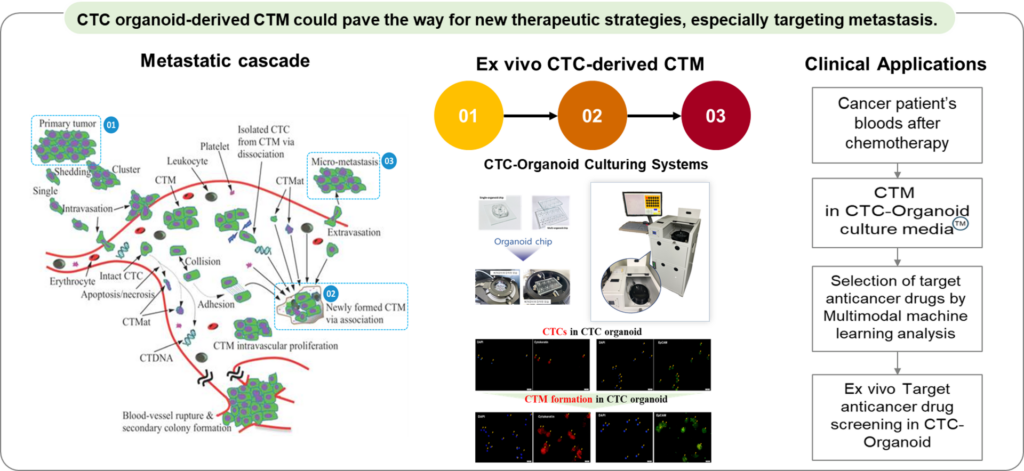
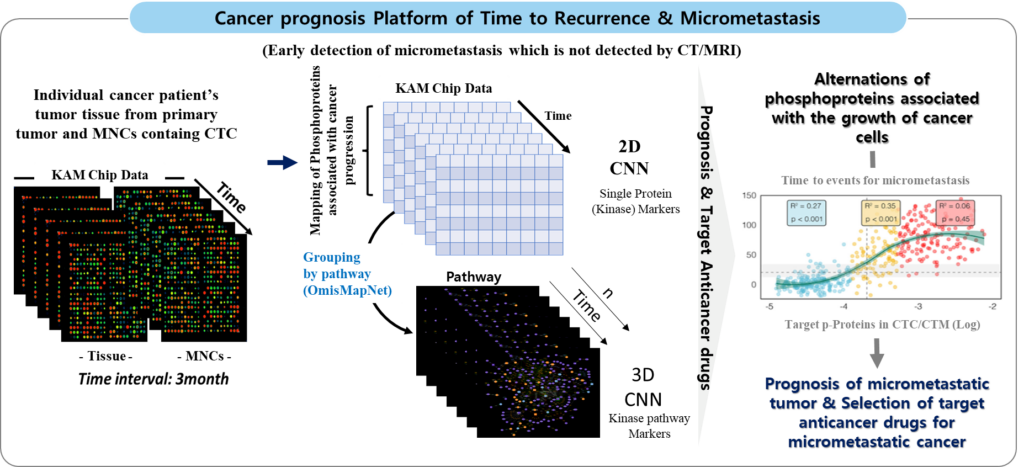
– Multimodal machine learning is a powerful tool for improving the precision and personalization of anticancer drug selection. By integrating multiple data modalities, it helps to provide more accurate and clinically relevant insights, potentially leading to better patient outcomes and more effective treatments. However, careful attention to data quality, interpretability, and validation in clinical settings is essential to ensure the successful application of these models.
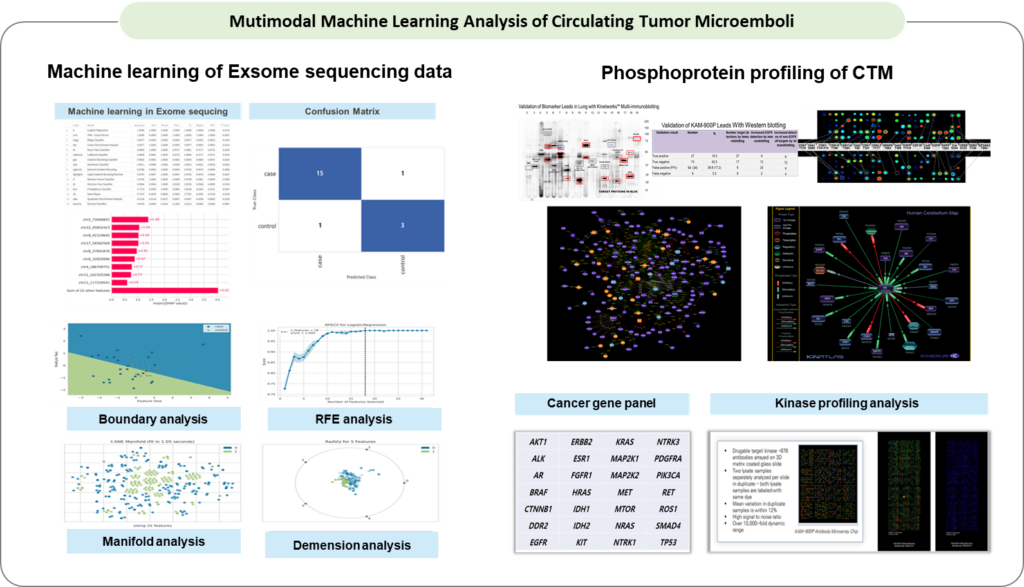
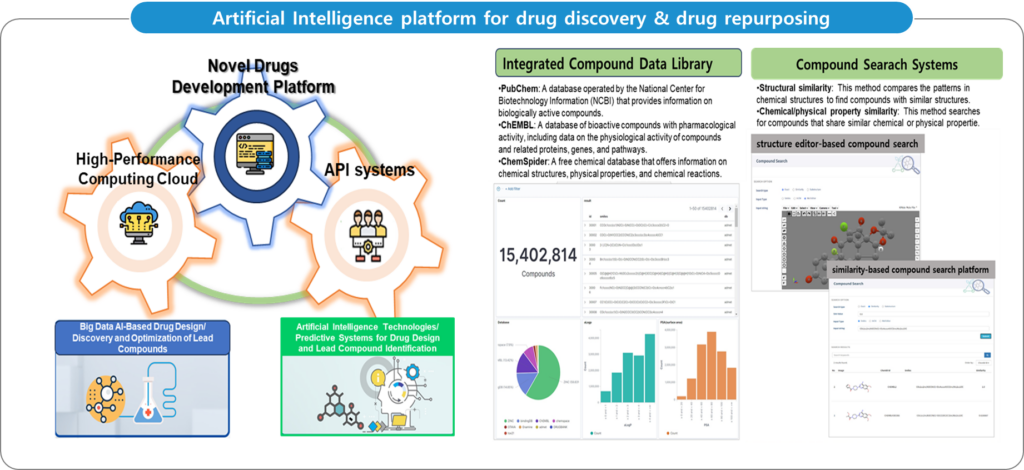